Do we Really Know that Chinese COVID-19 Statistics are being Manipulated?
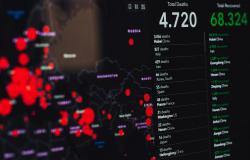
In all likelihood Chinese statistics on the pandemic’s spread are no worse – and no better -- than figures from Western democracies, suggest Roberto Aragão and Lukas Linsi.
For several weeks now global sports have been in lockdown. Stepping into the breach, a new pastime seems to have taken hold of news-hungry home-confined citizens: the following of coronavirus statistics. Facilitated by the attractive and user-friendly visualization of country-by-country infection and death rates in portals such as Johns Hopkins’ “Covid-19 map”, it has given rise to a novel, rather macabre, sort of international competition. Questions like “in which country did most people get infected today?”, or “are we still ahead of our neighbouring countries in terms of deaths?” have become hot topics at virtual cocktail parties and ‘Skypéros’. But this new sport is not only problematic on moral grounds. It also constitutes a largely meaningless exercise for mundane statistical reasons. The truth is that, for the most part, Covid-19 statistics are not informative indicators. And in particular they are not suited for cross-country comparisons.
The key figure of interest among followers of Covid-19 statistics is a country’s “case fatality rate”: the number of deaths in a country (the numerator) divided by the number of infections (the denominator). The resulting ‘league table’ ranks countries from the lowest rates – the places where governments and health authorities, so the story goes, have responded best to the crisis – to the highest. The problem is that both the numerator and denominator are biased in different directions in different countries. As a result, fatality rates are likely to be highly misleading when taken at face value.
Why is that so? Let’s start with the numerator. Counting the number of deaths due to Covid-19 infections is more complicated than it may sound. Establishing the cause of someone’s death can be hard, especially for patients suffering from several health problems simultaneously as well as for people who die outside of hospitals with little or no medical supervision. Because of such issues, authorities in different countries can count deaths differently. For instance, Covid-19 mortality statistics from some countries only include patients who passed away at a hospital, while others also include patients who deceased at home. Some countries only register a death as being caused by Covid-19 if the patient actually tested positive for the virus, whereas others do so if a patient showed Covid-19-like symptoms without having been tested for the disease. Likewise, in cases of multiple simultaneous medical conditions, some places indicate Covid-19 as the cause of death even if it may be due to a combination of factors; others don’t.
Moving from the nominator to the denominator, measurement problems become even more pronounced. This has to do with persisting uncertainties about the epidemiological character of the disease as well as well-known deficiencies in testing capabilities that most countries face. As of today, the number of asymptomatic cases (i.e. patients carrying the disease without showing symptoms) remains one of the great mysteries that epidemiologists trying to understand the rapid spread of the virus are trying to untangle. According to some studies the figure may be around 5 percent of total cases; according to others it may be as high as 80 percent. This means, in essence, that at present total actual infection rates (including symptomatic and asymptomatic) cannot be known with any certainty.
But we are not only uncertain about the extent of asymptomatic cases. Detection rates for symptomatic cases also vary dramatically across countries. This is primarily due to vast differences in countries’ testing strategies and capacities. A few small countries that test a lot (like Iceland) may be able to identify a good share of cases for which patients show symptoms. But in most countries detection rates are believed to be much lower. Even in a country with extensive testing like Germany the rate may lie well below 50 percent, while in developed countries with little testing, such as the Netherlands, the rate may be as low as 5 percent. So how many symptomatic infections may there be if say 10,000 cases have been detected in a country? The true figure may lie somewhere between 10,000-20,000 in a country carrying out extensive testing such as Germany, but anywhere between 10,000 and 200,000 in a country with little testing such as the Netherlands. In developing countries with less well-resourced health systems the true figure may be much higher still. If we take such issues into account, it seems clear that standardizing death rates by the number of detected cases and comparing them across countries makes little sense.
Cross-national differences in measurement practices are not unique to Covid-19 statistics. They are an intrinsic problem of most social indicators. In earlier research we have examined these same issues for highly established macroeconomic statistics with a proud history. Even there, we found that -- despite efforts by international organizations to harmonize statistics stretching back more than seven decades – significant cross-country discrepancies persist. In other words: even if Covid-19 statistics become somewhat better in the months and years to come as testing capacities improve, these problems will not disappear. Comparing them across countries is not meaningful today, and will remain fraught with difficulties in the future as well.
At a deeper level, the case of Covid-19 statistics is useful to illustrate an important general point about the politics of numbers: statistics are not mere reflectors of objective truths. They are socially and politically constructed concepts that are inherently ambiguous. As we have emphasized in our earlier research, recognizing the “softness” of numbers is crucial to better understand the politics of statistics. Not least, it pushes us to engage more critically with claims about “right” and “wrong” numbers – and, of particular importance in light of contemporary debates, claims about the manipulation of certain figures.
Debates about the manipulation of Chinese statistics
While everyone picks their own favourite when tracking Covid-19 statistics, most people in the Western world – ranging from the US President and the CIA to academics and the liberal press -- seem to be able to agree on at least one point: Chinese statistics on the spread of the virus are subject to political manipulation and hence particularly unreliable. As soon as the news that China added 1,290 deaths to the Wuhan death count hit the news this morning, commentators were quick to vindicate the statistical revision as proof of deliberate under-reporting as part of a general “cover-up” operation by the Chinese government.
Although we have no first-hand insights into the compilation of Covid-19 statistics in China, we have studied attempts by governments to manipulate economic statistics for several years. Based on our research findings, we have doubts about such assertions. As is the case for Covid-19 figures, macroeconomic statistics from China are also frequently singled out as being subject to political manipulation. As we found out, evidence supporting these claims is however not that strong for national-level economic data. While there is some evidence that subnational data from provincial authorities tend to overstate economic performance, the central governmental apparatus is highly aware of these dynamics and the Central Statistical Office uses a variety of methods to check and correct data submitted by subnational authorities, netting out most of these biases. While some studies still do indicate some remaining biases in national-level economic data, these are small in substantive terms, not unlike similar biases uncovered for a wide range of economic statistics observed in macroeconomic statistics from Western democracies. At least one study suggests that national-level economic data from China may even understate actual Chinese economic performance.
While Covid-19 statistics are different from macroeconomic data in some aspects, there are important parallels. Similar to economic statistics, both the theoretical and empirical case for large-scale manipulation is weak. Let’s unpack them one by one.
Theoretically, it remains unclear what a rational government in the situation that the Communist Party currently finds itself in would have to gain from obscuring the facts. Deliberately understating the seriousness of the situation would almost certainly foment the outbreak of a second wave of infections and a sharp increase in deaths. Attempts to hide a second outbreak would almost certainly prove futile as bodies pile up -- and very seriously undermine the legitimacy of the government. It seems altogether implausible that a rational government would take such risks simply to boast about its superior crisis management skills for a short while.
Empirically, it is almost certainly true that Chinese data is faulty. But that in itself is no proof of deliberate manipulation. China too grapples with the same serious measurement problems that Western democracies face. But when the Chinese government revises the death count upwards (as it did for Wuhan this morning) analysts are quick to shout “cover up” – irrespective of the fact that health authorities in Western democracies from New York, Italy, the UK among many others have done exactly the same thing over the past days and weeks without attracting such scrutiny.
To evaluate these intuitions somewhat more systematically, we conducted a ‘back-of-the-envelope’ calculation of the size of potential under-reporting in China using the open-access infection spread model developed by Nate Silver. For what they’re worth, the projections indicate that official Chinese case counts may under-estimate actual infection rates by about 75 percent. Now that is a substantial underestimation. However, it is relatively good in comparison to similar (and more rigorous) calculations made for some European countries by Neil Ferguson’s team at Imperial College. Their estimates suggest that – depending on the extent of asymptomatic infections – case counts from European countries may very well be under-reporting actual infection rates by more than 95 percent (and even 99 percent in the UK). So yes, Chinese case counts are likely to be far off, but they are no worse than those from liberal democracies.
The need for a scapegoat
Having studied data manipulation scandals in a variety of settings in depth over the past years, these dynamics are similar to what we have observed in many other instances as well. Most of the time, data manipulation scandals do not arise due to a sudden flagrant intervention with the production of statistics. They typically occur when it is in the interest of the accuser to see a data manipulation scandal dominating the news cycle. Contrary to their pretense to accuracy and objectivity, statistics have non-negligible error margins attached to them and they have various kinds of biases baked into them. But these biases typically only come to the fore in public debates when it is in someone’s interest to construct a data manipulation scandal. As we have argued, there are several indications that this time is no different.
We do not wish to whitewash Chinese statistics or the actions of the Chinese government. Chinese Covid-19 statistics are as imperfect as those of any other nation. There are serious questions that need to be asked about the role of the Chinese Communist Party in this crisis. But faulty numbers are far from being the most relevant one. Increasingly prominent claims in Western capitals that Chinese Covid-19 data is manipulated are not well founded. They are aimed at scapegoating more than anything else. Anyone claiming that Chinese numbers are the problem is trying to distract from what really matters: facing and finding ways out of an unprecedented global crisis.
Lukas Linsi is assistant professor of international political economy at the University of Groningen, Netherlands. Roberto Aragão is a PhD candidate at the University of Amsterdam, Netherlands. Both are affiliated to the Fickle Formulas research group.
Image: Markus Spiske via Pexels