Financial Trading Bots have Fascinating Similarities to People – We Need to Learn from Them
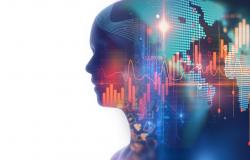
Christian Borch on why understanding how algorithms behave in crowds may be vital for our prosperity and safety.
In 2019, the world fretted that algorithms now know us better than we know ourselves. No concept captures this better than surveillance capitalism, a term coined by American writer Shoshana Zuboff to describe a bleak new era in which the likes of Facebook and Google provide popular services while their algorithms hawk our digital traces.
Surprisingly, Zuboff’s concern doesn’t extend to the algorithms in financial markets that have replaced many of the humans on trading floors. Automated algorithmic trading took off around the beginning of the 21st century, first in the US but soon in Europe as well.
One important driver was high-frequency trading, which runs at blinding speeds, down to billionths of a second. It offered investors the prospect of an edge over their rivals, while helping to provide liquidity to a market by ensuring there was always someone willing to buy and sell at a particular price. High-frequency trading is now behind more than half of the volumes in both the stock and futures markets. In other markets, such as foreign exchange, algorithms have a smaller but still significant presence, with no signs that they will wane in future.
The vices of devices
Humans still program the algorithms and design their trading strategies, though the rise of deep learning is putting even this role under threat. But the moment the algorithms go live on markets, they act on their own accord without human intervention, dancing with each other in dizzying and often unexpected ways.
At first glance, they have little in common with us. They cannot think or feel, and despite the hype around machine learning, it’s still contentious and complicated to describe them as intelligent. Like human traders, however, they make decisions, observe others making decisions, and adjust their behaviour in response.
At speeds many times faster than humans will probably ever muster, these algorithms easily form expectations about each other’s expectations when placing their buy and sell orders.
For example, one algorithm might seek to manipulate another’s expectations about price movements by sending a large number of orders to either buy or sell a particular asset. The first algorithm will then quickly cancel its orders, having hopefully tricked its rival into making the wrong bet about which way the market is heading.
Interestingly, sociologists consider this sort of mutual anticipation to be a central feature of what it means for humans to be social. They have long seen markets as highly social arenas. In the heyday of the trading floors, reading other traders’ social cues correctly – a grimace or grin, anxious tones, even the hubbub of the trading floor – often spelled the difference between wealth and disaster.
But if machines can be social, how similar or different is it to how humans socialise really? There are obvious differences, of course. While the human traders of the past often knew one another well, and often hung out together after work, algorithms trade anonymously. When they send orders to buy or sell assets, no other traders know whether it’s coming from a man or a machine.
Indeed, this is precisely why they are programmed to form expectations about one another. Facial cues are no longer available, but entire strategies have been developed that seek to find out whether a number of orders might have been placed by one and the same algorithm – and then try to predict what its next moves might be.
To evade such attempts, algorithms are often designed so as not to be recognised as algorithms by other algorithms. As the Scottish sociologist Donald MacKenzie has put it, they may engage in dissimulation strategies and/or seek to give a particular presentation of their “self” in public. These are again attributes that sociologists have long considered key aspects of metropolitan life.
Avalanche!
Together with colleagues, I have spent the past several years in major financial hubs interviewing traders, programmers, regulators, exchange officials and other finance professionals about these trading algorithms. This has drawn out some other interesting similarities between human and automated traders.
Programmers readily admit that once their algorithms start interacting with others, they get carried away and act unpredictably, as if they were in a mob. Sociologists since the late 19th century have studied how people get entranced by crowds and let their autonomy slide in “social avalanches”, but we have so far largely ignored the fact that financial machines do something similar.
The “flash crash” of May 6 2010 best illustrates what I mean here. In four a half minutes, the frenzied interaction of fully automated trading algorithms put the US markets into a nosedive, generating around US$1 trillion (£768 billion) of losses until trading was swiftly suspended.
Most of these trades involved were later cancelled as “clearly erroneous”. Certainly no trader or programmer had planned on creating this massive shift in prices, but decades of sociological research tell us that this sort of behaviour is expected in large groups. We need to understand how our financial algorithms interact in concert before our own tools become our undoing.
Of course, not all forms of social interaction are admirable or beneficial. Like humans, algorithms interact with each other in ways that range from caring and peaceful to cold and violent: from providing liquidity and maintaining market stability to making manipulative orders and triggering wild trading activity.
Getting to grips with these interactions is not only key to understanding modern trading and trying to prevent future flash crashes. Algorithms talk to one another in more and more fields today. Understanding how they behave as crowds will hopefully shed light in areas where they are just starting to come into their own – think self-driving traffic systems or automated warfare, for instance. It may even alert us to the avalanches that lie in wait, too.
Christian Borch, Professor of Economic Sociology and Social Theory, Copenhagen Business School.
This article is republished from The Conversation under a Creative Commons license. Read the original article.
Image: Automated for the people. WhiteMocca