AI Regulation in Practice: A View from the Private Sector
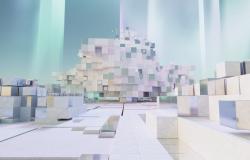
This is the second of two commentaries providing practice-based insights into how AI is being used, regulated and governed around the world. I had the pleasure of speaking with Till Klein of appliedAI, Europe's largest initiative for the application of trustworthy AI technology in companies, on December 16, 2022 regarding how AI is being put to work in the private sector.
The potential for artificial intelligence (AI) to improve business outcomes has been much lauded, particularly since the release of ChatGPT in November 2022and the subsequent proliferation of competitors. Promised benefits include enhanced flexibility, improved communication, greater productivity, and even enhanced customer satisfaction. Yet how does this work in practice? What challenges do businesses face in turning to AI? And how do political factors affect their capacity to achieve promised benefits?
I recently had the pleasure of speaking with Till Klein of appliedAI about these and related issues. appliedAI was created in 2017. Till notes that, at that time, it “was becoming really clear that there were new technological solutions to existing problems that were simply better than what we could access prior to that.” AI was increasingly being viewed as “a strategic asset of nations:” businesses use it to “improve productivity on a large scale” and this could increase prosperity for the nation. Yet it was also clear that Germany and Europe were far behind the advances taking place elsewhere in the world. So appliedAI set out to “help companies and other practitioners make use of AI for their own business” and in the process also maybe “expand Germany’s and the EU’s share of the pie.”
Challenges of AI in practice
The potentially transformative nature of AI at the business-level has led to a proliferation of advice regarding how businesses can optimize their gains from this technology. Till describes the decision to use AI as a journey. The first step of that journey is to commit to this “serious strategic initiative [which] is not going to have returns on the first day” and which requires substantial effort to realize. Many companies begin as “experimenters,” trying out a single application to see how it performs and how it affects their business. As their confidence and experiences grow, they become practitioners, systematically analyzing how use cases interact and how this “creates new synergies” which are advantageous for their business. Practitioners develop AI strategies addressing their individual business needs, for instance, by creating “systematic education programs for people working at the company to build up skills” or building a “data strategy” to ensure access to good data for AI training purposes. Companies continuing along this path ultimately become very professional in their use of AI, adopting “almost an AI-first approach” where they respond to new challenges by asking “how can we use AI for that?”
While this seems straightforward, Till underlines that there are several hurdles which companies tend to face when proceeding along this path. A first challenge involves determining the use case that is most likely to yield benefits in a given setting. This is a “non-trivial task” as businesses may only invest in one use case and want to be sure they’re “putting their eggs into the right basket.” appliedAI suggests a framework for thinking about use cases based on ideation, assessment, prioritization and execution. In the ideation phase, businesses ask questions like, “should I focus on internal processes like production and finance and logistics or should I be focusing on the external facing activities like customer touchpoint, customer interaction, new offerings?” These ideas are then assessed via internal processes or in collaboration with partners like appliedAI. Once a business has determined its priorities in using AI, it then moves into the execution phase to begin reaping rewards from AI.
(Brakemeier et al 2023)
Another set of challenges arises at this stage. Till notes common ones include evaluating and enhancing staff capacity to implement AI solutions and making structural or strategic shifts which may be necessary for successful implementation. For instance, “Is AI an IT topic? Should we create a dedicated AI unit?” These questions are often difficult to answer, even for digitally advanced and/or larger firms. For one thing, incorporating technology in business activities, which companies have done for many years, “is different to developing AI solutions.” To effectively use AI, you may need “to rethink your development pipeline and the skills of the people involved,” which can have “implications for commercial goals and strategies” as well. Moreover, Till notes that studies have shown that “building up AI capability is one of the main entry barriers for using AI.” The AI talent pool is a “very competitive market with very high salary packages” with “large companies in Germany, like the automotive ones, competing against the big tech players from overseas who have offices in Europe as well.” This limits the capacity of many firms to address AI skill deficits via recruitment. Yet the more affordable option of relying on collaboration with external vendors has its costs as well. For instance, “if I use the I system from a vendor build a competitive edge, anyone else can buy it as well and there goes my competitive advantage.”
Enter the political context
Till was quick to note that businesses do not use AI technologies in a vacuum. AI is “a technology that is locally developed and globally deployed” with “the main models coming from a very few players overseas, mostly big tech in the US and China, with this whole nation or winner-takes-all mentality.” As he sees it, these firms “just had a bit of an advantage, like the Google search for instance, at some point in time, but then there’s this flywheel effect: because it’s better at one time, people use it and it generates more data, helping you to learn faster. Like so much better than everyone else, that it’s really difficult to catch up. That creates inequalities for everyone else.” These tendencies can be countered. For example, appliedAI uses targeted education programs and provides information to the general public on AI technologies and their uses to “make sure that AI is not only for those who can afford to hire the people, but also for everyone else.”
Yet a supportive regulatory context matters as well. Till notes that, “by and large, AI regulations are welcome because, in the long run, they create clarity. It’s like moving from the Wild West to some organized setting, establishing mutual expectations of what can and cannot be done while also shaping the ecosystem.” Nonetheless, debates in Brussels tend to be “a lot of talk about big tech from overseas, what Facebook and Alibaba have done and geopolitical tensions. We should definitely consider this, but we should not overlook the local AI system, the innovation and startups coming from our universities across Europe.” To that end, appliedAI and others “inform the negotiations from a practical perspective,” generating insights from “people or organizations developing AI” and communicating these via white papers and studies. These communities provide “empirical insights” into what it would cost to be compliant with proposed regulations and how they will be affected by propositions like the European Commission’s risk-based approach to classifying AI systems. The hope is that more useful regulations will be the result.
Here too Till underlines a need to keep in mind that implementing regulations like the proposed AI Act – much like the AI technologies they seek to regulate – is a process. “In the short term, it will increase the complexity of something that is already extremely complex. It will probably take us a couple of years to establish dominant methods for how to be compliant and this will slow us down.” Yet he notes experience suggests the end result will ultimately be better. When seat belts were introduced, “auto manufacturers had to upgrade and their developers were saying, ‘Oh no, new rules, new this, new that,’ but now clearly no one would ever use a car without a seat belt.” He thinks this can be compared to where Europe is with AI at the moment: people are hesitant because of the turmoil but, in a few years, “they have this regulatory framework where they feel comfortable and maybe it will be more worth the investment than it previously was. And then we’ll be happy and look back into this turmoil we have right now and say, it was worth the hassle to enjoy good quality” arising from businesses’ investments in AI technology.
Till Klein is the Head of Trustworthy AI at the appliedAI Initiative, a leading initiative of applied AI in Europe, where he is working toward accelerating Trustworthy AI at scale. He has several years of practical experience with Regulations in High-Tech sectors through roles in Regulatory Affairs for Medical Devices, as Lead Auditor for ISO 9001, and as Head for Quality Management in a Drone Company. Till is an industrial Engineer by training and holds a Ph.D. (Business) on Technology Transfer and Collaboration Networks from the Swinburne University of Technology in Melbourne, Australia.
Laura Mahrenbach is an adjunct professor at the School of Social Sciences and Technology at the Technical University of Munich. Her research explores how global power shifts and technology interact, with a special focus on the implications of this interaction for the countries of the Global South as well as related governance dilemmas at the national and global levels. More information available at www.mahrenbach.com. This work was supported by Deutsche Forschungsgemeinschaft (Grant No. 3698966954).
Photo by Google DeepMind